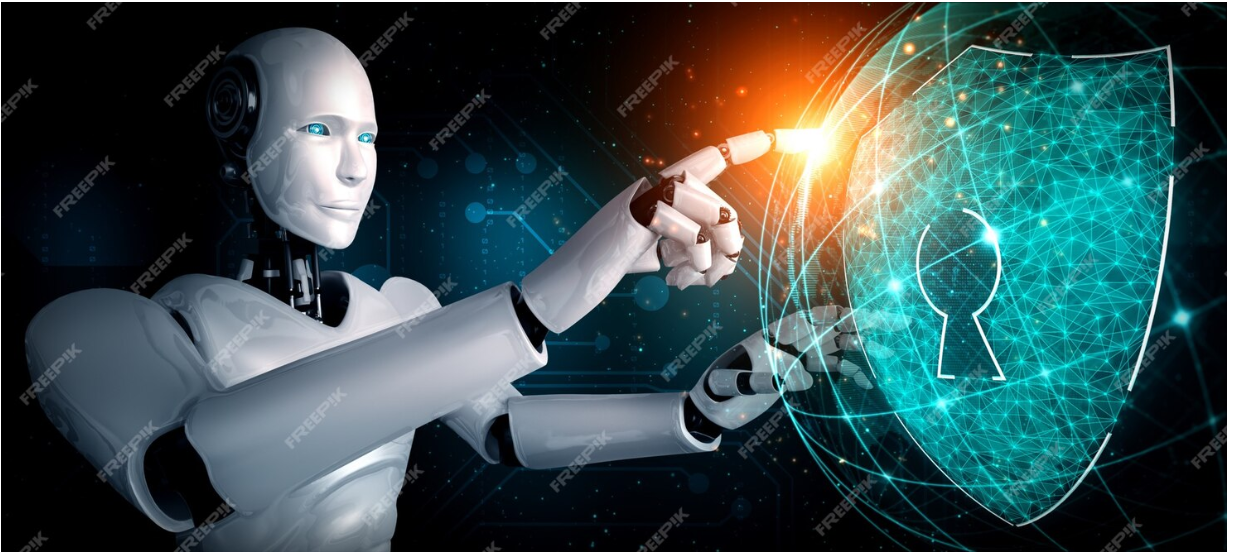
How Artificial Intelligence is Transforming Cybersecurity
Posted on May 4, 2024
How Artificial Intelligence is Transforming Cybersecurity
In recent years, artificial intelligence (AI) has emerged as a powerful tool in the fight against cybercrime. As cyber threats become increasingly sophisticated, traditional security approaches are often inadequate in identifying and responding to potential threats in real time. By leveraging AI, cybersecurity professionals can stay ahead of attackers, enhancing detection capabilities, automating threat response, and providing a more robust defense against complex cyber threats. This blog explores how AI is transforming cybersecurity and shaping the future of digital defense.
The Growing Need for AI in Cybersecurity
The modern digital landscape is vast and rapidly expanding, with more devices connected to the internet than ever before. This digital proliferation creates a larger attack surface for cybercriminals. Every day, thousands of new malware strains are developed, phishing scams are executed, and data breaches occur. Traditional cybersecurity measures, often reliant on human intervention and predefined rules, struggle to keep up with these emerging threats.
AI, with its ability to analyze massive amounts of data and recognize patterns, offers a solution. By automating the process of threat detection and response, AI can help cybersecurity teams identify and address vulnerabilities faster and more accurately than manual methods.
Key Ways AI is Transforming Cybersecurity
Let’s look at some of the transformative ways AI is enhancing cybersecurity:
1. Improved Threat Detection with Machine Learning
One of the most significant benefits of AI in cybersecurity is its ability to detect threats in real time. Traditional methods rely on known threat signatures to identify malware or malicious activity. However, this approach falls short when dealing with new or unknown threats, often called zero-day attacks.
AI-powered threat detection uses machine learning (ML) to analyze vast datasets, identifying unusual behavior that may indicate an attack. By continuously learning from this data, AI models become better at recognizing subtle patterns and can spot potential threats before they become full-fledged attacks.
Example: Darktrace, an AI-driven cybersecurity company, employs machine learning algorithms to detect subtle shifts in network behavior that may signal a breach, allowing organizations to respond immediately.
2. Enhanced Phishing Detection
Phishing remains one of the most common forms of cyberattacks, often exploiting human error to steal sensitive information. Traditional spam filters rely on rule-based systems, which can miss sophisticated phishing attempts that do not match established patterns.
AI enhances phishing detection by analyzing email content, metadata, and sender behavior to spot suspicious emails more accurately. Natural language processing (NLP), a branch of AI, can examine the language used in emails to detect subtle signs of phishing, even if the message appears legitimate at first glance.
Example: Google uses AI to filter spam and phishing emails in Gmail, blocking billions of malicious messages daily. This system constantly improves, identifying new forms of phishing as they emerge.
3. Automated Threat Response and Incident Management
Manual threat response can be slow and resource-intensive, giving cybercriminals time to cause extensive damage. AI enables faster response times by automating threat containment and remediation processes. With Security Orchestration, Automation, and Response (SOAR) tools, AI can automatically isolate infected systems, stop suspicious processes, and block unauthorized access.
Automating these responses saves time, reduces the risk of human error, and allows cybersecurity teams to focus on more complex tasks that require human insight.
Example: An AI-driven system can detect a ransomware attack in its early stages, isolate the affected computer, and prevent the spread of ransomware across the network.
4. Behavioral Analysis and Anomaly Detection
AI excels at monitoring user behavior and network traffic to identify unusual patterns that could signal a security threat. By establishing a baseline of “normal” activity, AI can detect deviations that indicate suspicious behavior.
For example, if an employee’s login pattern suddenly changes or if there is an unusual spike in data downloads, AI can flag these activities as potentially malicious. This approach is particularly useful for detecting insider threats and compromised accounts.
Example: A financial institution might use AI-driven behavioral analysis to monitor employees’ access to sensitive data. If an employee suddenly starts accessing large volumes of confidential information, the AI system could alert security teams to investigate.
5. Predictive Analytics and Threat Intelligence
Predictive analytics is a powerful tool in AI, allowing cybersecurity teams to anticipate future threats based on historical data. By analyzing past incidents, AI can identify patterns that often precede cyberattacks, such as certain types of suspicious activity or increased probing by unauthorized users.
Threat intelligence gathered by AI can help organizations stay one step ahead of cybercriminals by preparing for likely attack vectors. This proactive approach can prevent incidents before they occur, rather than merely reacting to attacks as they happen.
Example: Predictive AI tools can identify indicators of an impending Distributed Denial of Service (DDoS) attack and allow security teams to deploy protective measures before the attack occurs.
6. Strengthening Identity and Access Management (IAM)
Traditional password-based authentication methods are increasingly inadequate for today’s cybersecurity needs. AI-driven Identity and Access Management (IAM) solutions enhance security by continuously analyzing user behavior and adapting authentication requirements accordingly.
For instance, AI can trigger additional security checks for a user exhibiting unusual login behavior, such as logging in from a new device or an unfamiliar location. This approach, known as adaptive or risk-based authentication, reduces the reliance on passwords alone, making unauthorized access more difficult.
Example: Banks use AI-powered IAM systems to authenticate users based on behavioral biometrics, like typing speed and navigation patterns, making it harder for hackers to impersonate legitimate users.
7. AI-Powered Vulnerability Management
Identifying and patching vulnerabilities is critical to preventing cyberattacks, but it’s also time-consuming and complex. AI can automate vulnerability management by scanning systems for weaknesses, prioritizing them based on risk, and even suggesting or applying fixes.
AI-driven tools can help organizations manage their patching schedules more efficiently, reducing the risk of known vulnerabilities being exploited by attackers.
Example: Some cybersecurity companies offer AI-based solutions that continuously scan software and network systems, alerting administrators to unpatched vulnerabilities and automatically applying updates.
Challenges of Implementing AI in Cybersecurity
While AI brings immense benefits to cybersecurity, it also comes with certain challenges:
- Data Privacy: AI systems often require large amounts of data to train effectively, raising concerns about data privacy and regulatory compliance.
- False Positives: AI algorithms may occasionally flag harmless activity as a threat, leading to unnecessary alerts and potential disruption.
- Skill Gap: Implementing AI requires specialized skills and knowledge, and finding qualified professionals can be challenging for some organizations.
- Risk of AI-Powered Attacks: Cybercriminals are also using AI to enhance their tactics, creating a continuous “cat-and-mouse” game between attackers and defenders.
Despite these challenges, AI continues to be a vital asset in cybersecurity, with ongoing advancements improving its accuracy, reliability, and ease of integration.
The Future of AI in Cybersecurity
As AI technology advances, we can expect it to play an even more prominent role in cybersecurity. Future applications may include:
- Enhanced Threat Intelligence: Using AI to analyze global threat data in real time, providing insights into emerging threats and evolving attack techniques.
- Automated Security Systems: Fully autonomous systems that can detect, respond to, and neutralize cyber threats with minimal human intervention.
- Advanced Behavioral Biometrics: AI-driven biometric authentication that relies on a user’s unique behavioral patterns, such as mouse movements, voice patterns, and facial recognition.
- AI-Augmented Cybersecurity Teams: Instead of replacing human cybersecurity professionals, AI will increasingly serve as an invaluable ally, allowing teams to focus on strategic tasks and complex decision-making.
Conclusion
Artificial intelligence is revolutionizing cybersecurity, providing organizations with powerful tools to defend against today’s complex cyber threats. By automating threat detection, enhancing response capabilities, and enabling predictive analytics, AI empowers cybersecurity teams to act faster and more efficiently than ever before. While challenges remain, the benefits of AI-driven cybersecurity are undeniable, and as technology continues to evolve, AI will play an even more crucial role in safeguarding the digital world. Embracing AI in cybersecurity is not just an advantage but an essential strategy for protecting sensitive data, maintaining customer trust, and staying resilient in the face of a dynamic threat landscape.