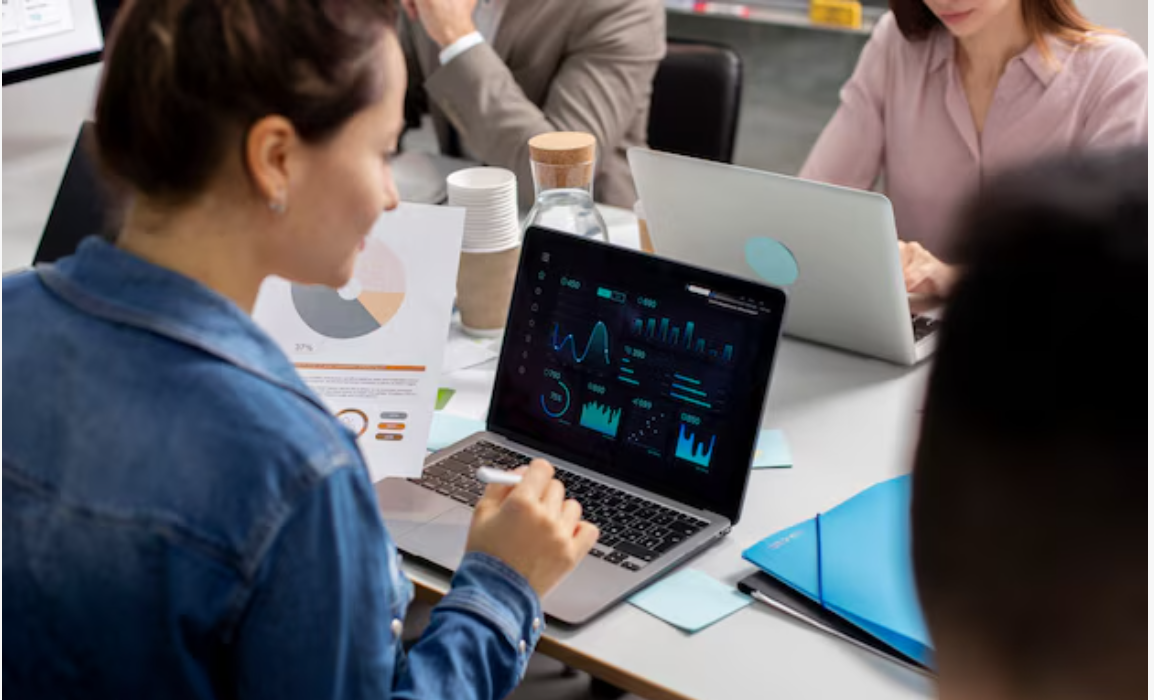
The Role of Machine Learning in Predictive Analytics for Business
Posted on April 4, 2024
In today’s data-driven landscape, businesses are inundated with vast amounts of data generated from various sources. To navigate this ocean of information and extract actionable insights, companies are increasingly turning to predictive analytics powered by machine learning. This blog delves into the transformative role of machine learning in predictive analytics, exploring its key concepts, benefits, applications, and challenges.
Understanding Predictive Analytics
Predictive analytics is a branch of advanced analytics that uses historical data, statistical algorithms, and machine learning techniques to identify the likelihood of future outcomes. By leveraging patterns found in historical data, businesses can make informed decisions and strategically position themselves for success. Predictive analytics can be applied across various domains, including finance, marketing, healthcare, and supply chain management.
Machine Learning: A Key Enabler
Machine learning (ML) is a subset of artificial intelligence (AI) that enables systems to learn from data and improve their performance over time without being explicitly programmed. By utilizing algorithms that can process and analyze large datasets, machine learning enhances predictive analytics in several ways:
- Data Processing and Analysis: Machine learning algorithms can handle massive amounts of data quickly and efficiently, uncovering patterns and trends that may not be apparent through traditional analytical methods. This capability is crucial for businesses that generate large volumes of data daily.
- Enhanced Accuracy: Machine learning models can continuously learn and adapt to new data, leading to improved accuracy in predictions. As more data is fed into the system, the model refines its understanding of the underlying patterns, resulting in more precise forecasting.
- Automation: By automating the data analysis process, machine learning reduces the need for manual intervention, enabling businesses to scale their predictive analytics efforts. This automation frees up human resources to focus on strategic decision-making and other high-value tasks.
- Real-time Insights: Machine learning algorithms can analyze data in real-time, allowing businesses to make timely decisions based on the most current information. This agility is particularly valuable in fast-paced industries where market conditions can change rapidly.
Applications of Machine Learning in Predictive Analytics
The integration of machine learning into predictive analytics has far-reaching applications across various industries. Here are some notable examples:
- Customer Behavior Prediction: Businesses can analyze customer data to predict purchasing behavior, preferences, and churn rates. By understanding these patterns, companies can tailor their marketing strategies, improve customer retention, and enhance the overall customer experience.
- Risk Management: In finance and insurance, predictive analytics powered by machine learning can assess the likelihood of defaults, fraud, or claims. This allows organizations to mitigate risks effectively and make informed lending or underwriting decisions.
- Supply Chain Optimization: Machine learning algorithms can predict demand fluctuations, enabling businesses to optimize inventory levels, reduce waste, and enhance supply chain efficiency. This is particularly important in industries with volatile demand, such as retail and manufacturing.
- Healthcare Outcomes: In healthcare, predictive analytics can identify potential health risks, predict patient outcomes, and optimize treatment plans. Machine learning models can analyze patient data to detect patterns that indicate the likelihood of diseases or complications, improving patient care and resource allocation.
- Marketing Campaign Effectiveness: Machine learning can analyze historical campaign data to predict the success of future marketing initiatives. By understanding which channels and messages resonate with target audiences, businesses can optimize their marketing strategies and maximize ROI.
Challenges in Implementing Machine Learning for Predictive Analytics
Despite its numerous benefits, the implementation of machine learning in predictive analytics is not without challenges:
- Data Quality: The effectiveness of machine learning algorithms relies heavily on the quality of the data used for training. Incomplete, inconsistent, or biased data can lead to inaccurate predictions and flawed decision-making.
- Complexity: Machine learning models can be complex, making them difficult to interpret. This complexity can hinder transparency and trust among stakeholders, particularly in industries like finance and healthcare, where decisions based on predictive analytics can have significant consequences.
- Resource Intensive: Developing and deploying machine learning models require significant computational resources and expertise. Organizations may face challenges in recruiting skilled data scientists and analysts who can effectively build and maintain these systems.
- Ethical Considerations: As with any AI application, ethical considerations surrounding data privacy and bias must be addressed. Organizations must ensure that their predictive analytics practices comply with regulations and do not inadvertently reinforce biases present in historical data.
Conclusion
The integration of machine learning into predictive analytics is revolutionizing the way businesses operate. By harnessing the power of data, organizations can make informed decisions, anticipate future trends, and gain a competitive edge in their respective markets. While challenges exist, the potential benefits of machine learning in predictive analytics far outweigh the obstacles, making it a critical component of modern business strategy. As technology continues to evolve, the future of predictive analytics will likely be shaped by even more advanced machine learning techniques, further enhancing its impact on business decision-making.
By embracing these advancements, companies can position themselves for success in an increasingly complex and data-driven world.